HiTS Research Summary
Improving treatment options for patients with serious diseases such as cancer and neurodegeneration is critically dependent on stratifying disease based on molecular mechanisms. This is challenging because many diseases - as classically defined - have a variety of causes, each of which requires a different “precision” medicine approach. In the last two decades, genomics has provided the bulk of the data used for disease stratification but its strengths and limitations are becoming increasingly clear. Future discoveries will require integrating genomics with rigorous quantitative analysis of the many other types of data – images for example – that are currently subjected only to human interpretation. HiTS is dedicated to combining sophisticated measurement methods with machine learning and artificial intelligence to greatly accelerate pre-clinical and translational drug discovery. We are also actively studying the design and interpretation of clinical trials with the goal of reducing the high rate of late stage failure.
HiTS specializes in projects that span biomedicine, engineering, and computer science and engage faculty, students and fellows with a range of skills, typically from multiple institutions. We also prioritize the development and maintenance of new software and the invention of fundamentally new approaches to pre-clinical and clinical pharmacology. Individual projects focus on diseases including drug resistant cancers, infectious disease, neurodegenerative disease and chronic pain and a wide range of drug types from small molecules to modified peptides, antibodies and cells. At the same time, most projects have strong basic science components rooted in better understanding of cellular networks and homeostatic mechanisms. Key projects include the following:
Areas of Research
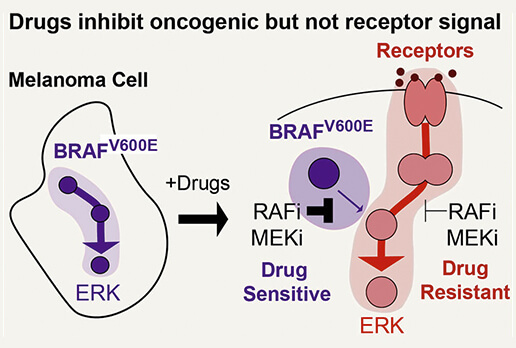
Identifying the Determinants of Sensitivity and Resistance to Small Molecule Kinase Inhibitors
Kinase inhibitors are an intensively studied class of therapeutics with many remaining unknowns – LSP investigators use them to develop new approaches for identifying targets and to study the factors determining drug resistance and sensitivity.
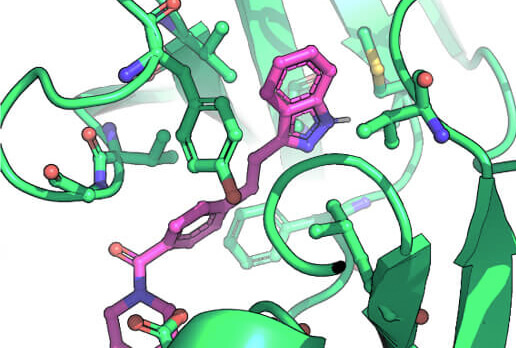
Using Deep Learning to Model Drug-Target Engagement
LSP investigators are contributing to and benefiting from the rapid advances in deep learning that are revolutionizing data analysis, protein structure prediction, and our ability to invent new drugs.
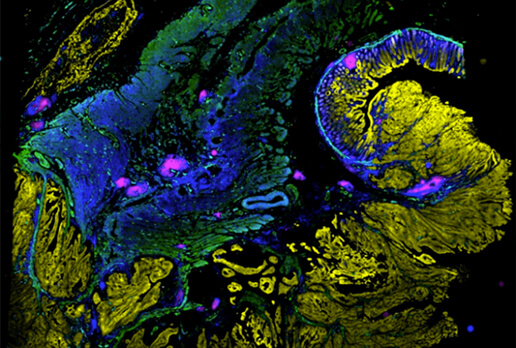
Developing next generation diagnostics and immunoprofiling methods using digital pathology and AI
The LSP has developed new highly multiplexed imaging methods and computational tools that enable deep molecular interrogation of tumor-immune interactions and the development of next-generation diagnostics for precision cancer care.
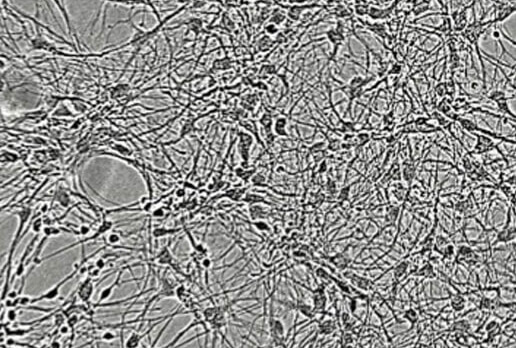
Repurposing Drugs to Treat Alzheimer’s Disease
LSP investigators working with the Massachusetts Alzheimer's Disease Research Center at the MGH are developing new approaches to treat Alzheimer's Disease based on the hypothesis that the disease has multiple distinct etiologies, some involving degeneration-associated chronic inflammation.
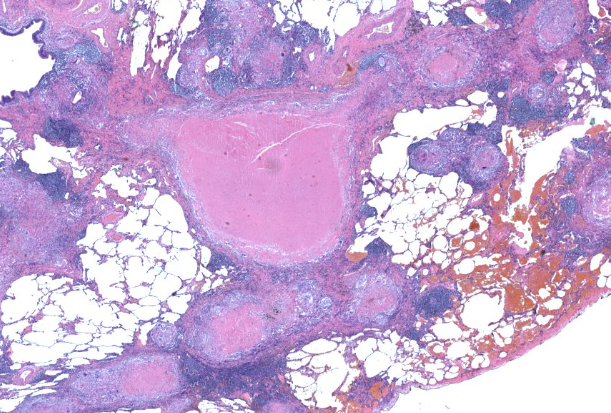
Developing Cures for Tuberculosis
Through a close collaboration with Tufts Medical School, LSP scientists are using computational approaches, animal models, and deep profiling of TB granulomas to understand how mechanisms of combination therapy and improve treatment approaches.
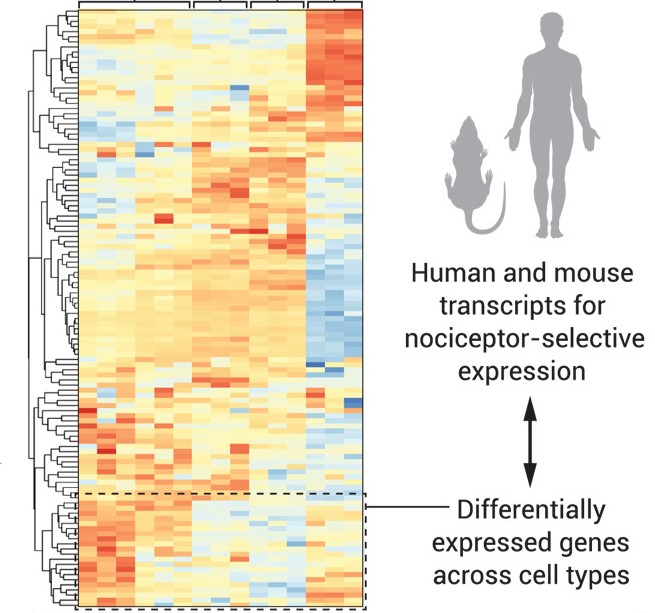
Identifying New Non-Opioid Therapeutics for Pain
LSP investigators are developing novel non-opioid treatments for pain with greater long-term efficacy and lower potential for abuse using screens in iPSC-derived neurons, cheminformatics, and animal models.
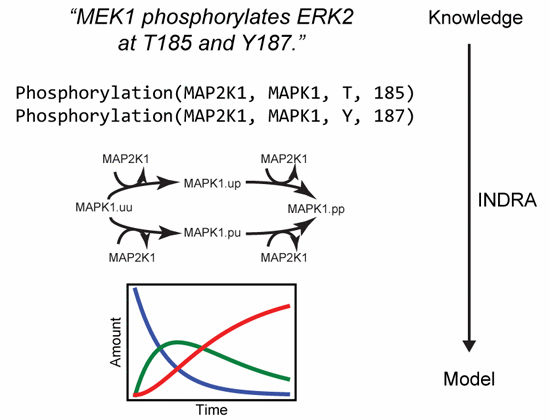
Using Knowledge Assembly to Enable Network Biology
The LSP is developing new computing platforms and knowledge assembly systems to dramatically improve how we extract causal and mechanistic information from published literature.
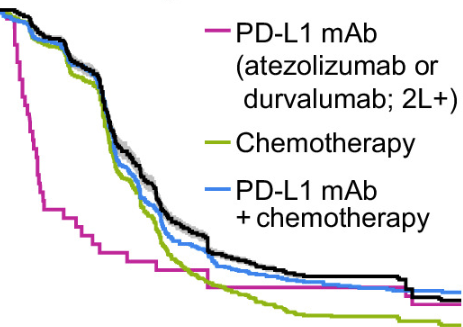
Improving the Performance and Interpretation of Cancer Trials
The LSP is engaged in a wide-ranging project to understand the causes of success and failure in cancer clinical trials and develop improved computational methods for early-phase trials that can help predict trial success.